
Platform: UserZoom
Innovation fueled by UX insights
UserZoom empowers anyone to create digital experiences that delight customers and drive business growth.
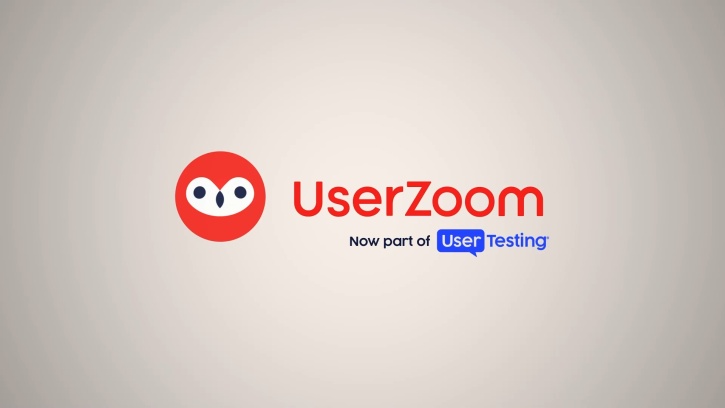
Why UserZoom?

Capture human insight throughout the product development lifecycle. Our comprehensive testing solution adapts to meet your team’s testing needs.

Quickly identify key insights and measure performance with analytics and visualizations designed to save time and simplify post-test analysis.

Design experiences your users love through a deep understanding of their wants, needs, and behaviors.

Quickly capture high-quality, diverse perspectives from the UserTesting Network, partner networks, and your own network. Define your audience and choose your sample size. We’ll handle the rest.

The confidence of knowing you have enterprise-level security with our SOC 2 Type II certified, GDPR, and HIPAA-compliant platform.
How it works
Design your study
Leverage the widest range of testing capabilities on the market—test everything from ideas, designs, and prototypes to websites, campaigns, and competitive brands. Mix and match usability testing, surveys, information architecture testing, and more for unparalleled flexibility.

Recruit your target audience
Quickly fill your studies with top-quality participants from a global network of participants who have opted in to provide feedback. Our machine-learning-powered distribution engine helps you find the right audiences with over 200 demographic filters for any type of test.
You can also invite your audiences through live intercepts or share a custom study link via email, text, Slack, and social media.

Seamlessly launch and schedule studies
UserZoom will automatically send study invites and reminders to participants. For moderated studies, invite your team to collaborate during sessions from a virtual observation room–completely out of sight from the participant.

Get automated insights
Accelerate your analysis with automated transcripts, synced playback, timestamped notes, and auto-generated charts and visualizations.

Evangelize insights
Use video clips, highlight reels, and quotes to create engaging and impactful user stories with your insights and empower your team with the data they need to make quick, confident decisions.

Pricing to scale with your business
Whether you’re an early-stage startup or an established enterprise business, we have the right plan to meet your needs and scale research across global teams as your business grows.

Solutions for every team

Make user research faster, easier, and more inclusive for your entire organization.

Gather feedback, validate designs, and move projects forward quickly and efficiently.

De-risk and prioritize product investment decisions with confidence.

Improve digital experience, campaign, and brand performance.
Insights that drive business outcomes
The world’s most innovative companies trust UserZoom
The combination of both rapid and qualitative research with remote testing at scale and quantitative metrics provides statistical significance for stakeholders.
UserZoom is the high-performance supercar of research platforms. And you're just an incredible partner too. People are excited to use the tool and then they're also excited to get to talk to your UX research experts.
UserZoom's research tools have transformed our delivery of insight, and our roles would be virtually impossible to perform without them. Outputs from our UserZoom testing help us positively impact the lives of our customers every day.